Unlocking the Power of Image Labeling Tools for Object Detection
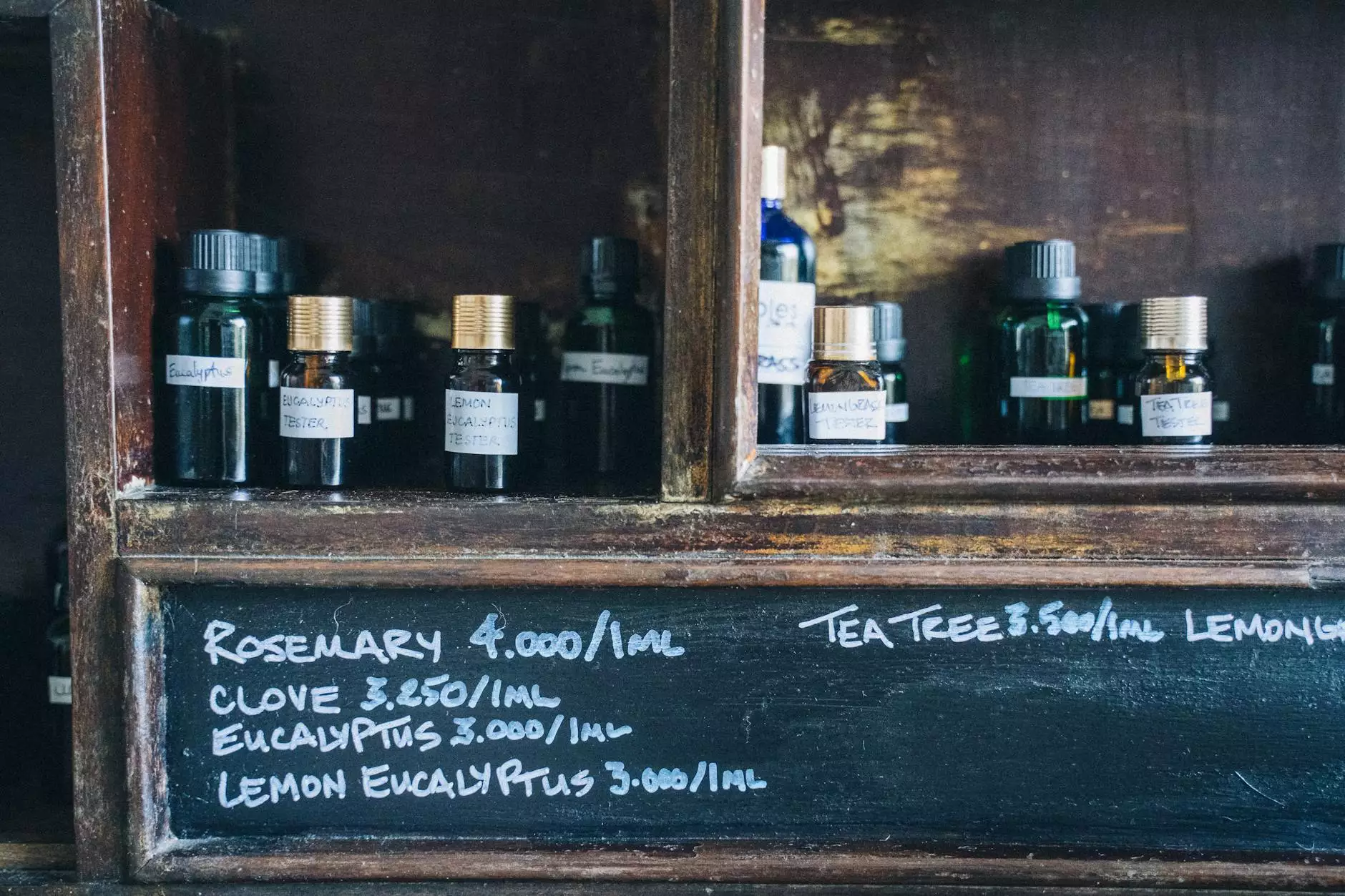
In the realms of machine learning and computer vision, the significance of accurate data cannot be overstated. As we venture deeper into the digital age, image labeling tools for object detection have emerged as pivotal resources for businesses looking to harness the potential of AI-driven solutions. At the forefront of these tools is Keylabs.ai, where we empower organizations with robust data annotation tools and a comprehensive platform for all their annotation needs.
Understanding Image Labeling Tools
Image labeling tools are sophisticated software solutions that automate and expedite the process of annotating images. Annotation is crucial in training machine learning models, particularly for tasks such as object detection, image segmentation, and facial recognition. By providing humans with the ability to label images accurately, these tools enable machines to interpret visual data similarly to how we do.
The primary objective of image labeling tools for object detection is to make it easier for organizations to create datasets that can effectively train AI algorithms. A well-annotated dataset improves the AI model’s accuracy, leading to better performance in real-world applications.
The Necessity of Data Annotation in AI
Data annotation is the foundation of machine learning. High-quality, well-annotated datasets are essential for building reliable AI systems. Here’s why data annotation is crucial:
- Enhances Model Performance: Accurately annotated data leads to improved predictions and classification.
- Facilitates Supervised Learning: Image labeling allows AI to understand the context and dynamics of various objects in images.
- Reduces Bias: A diverse and comprehensive dataset aids in minimizing bias in AI outputs.
- Boosts User Trust: Well-annotated data increases the credibility of AI systems among users.
Key Features of Effective Image Labeling Tools
When selecting an image labeling tool for object detection, there are several key features to consider:
1. User-Friendly Interface
A tool with an intuitive, user-friendly interface enables annotators to work more efficiently, minimizing the learning curve and enhancing productivity.
2. Support for Multiple Annotation Types
Different projects may require different types of annotations. A top-tier labeling tool should support bounding boxes, polygons, image segmentation, and more.
3. Automation Capabilities
Automation features such as pre-annotations using AI can significantly reduce the time spent on manual labeling and improve consistency.
4. Collaboration Tools
The ability to collaborate in real time is essential, particularly for large projects that involve multiple stakeholders.
5. Quality Assurance Mechanisms
Integrated quality checks and review stages ensure that the labeled data meets the necessary standards for accuracy and reliability.
How to Choose the Right Image Labeling Tool
Selecting the right image labeling tool for object detection involves several factors:
- Project Requirements: Understand the specific needs of your project, such as the type of objects to be detected and the quantity of images to be annotated.
- Budget: Consider the costs associated with different tools and platform subscriptions.
- Scalability: Choose a tool that can grow with your project’s needs as they evolve.
- Integration: Ensure the tool can easily integrate with other technologies and platforms you are using.
Business Applications of Image Labeling Tools
The applications of image labeling tools for object detection span numerous industries. Here's a closer look at how businesses implement these tools:
1. Retail and E-Commerce
In the retail sector, companies utilize image labeling to enhance inventory management through object detection systems—labeling products in images allows automatic tracking and categorization of inventory.
2. Autonomous Vehicles
Self-driving cars rely heavily on image labeling to identify and react to various objects in their environment, such as pedestrians, other vehicles, and road signs.
3. Healthcare
In the healthcare field, image annotation aids in identifying and classifying medical conditions through imaging analysis, enabling better diagnosis and treatment plans.
4. Agriculture
Farmers are leveraging image labeling tools to monitor crop health and detect pests, diseases, or yield predictions using aerial or drone imagery analysis.
The Data Annotation Process with Keylabs.ai
At Keylabs.ai, we take a meticulous approach to data annotation, ensuring that every image is accurately labeled for optimal machine learning results. Here’s a brief overview of our process:
Step 1: Project Assessment
The first step involves a consultation to understand your specific requirements, including the types of objects to label and the overall goals of your project.
Step 2: Dataset Preparation
We help you prepare your dataset, ensuring it is suitable for the specific annotations required for your project.
Step 3: Annotation Execution
Using our advanced image labeling tools, our team of skilled annotators will meticulously label each image to meet your specifications.
Step 4: Quality Assurance
We implement rigorous quality control processes to ensure the highest accuracy of labeled data before it is delivered to you.
Step 5: Delivery and Feedback
Once the annotations are complete, we provide the labeled data in your desired format and remain open to feedback to ensure total satisfaction.
Conclusion
In conclusion, the significance of image labeling tools for object detection in today's data-driven landscape cannot be overstated. By investing in high-quality annotation tools and platforms like Keylabs.ai, businesses can greatly enhance their machine learning capabilities, leading to improved operational efficiencies and innovative solutions across myriad industries.
For those looking to dive into the world of AI, data annotation is a crucial step. Embrace the power of image labeling tools and watch as your organization thrives in the competitive landscape of the digital age.